Innovation Accelerated
At Describe Data, we provide bespoke consulting services that integrate advanced technology expertise with insurance business acumen.
Our approach is to balance technology with pragmatism, working closely with client actuaries, underwriters and technologists.
From strategic guidance to implementation, we are committed to helping our clients to navigate complexity, enhance operational efficiency, and drive sustainable growth.
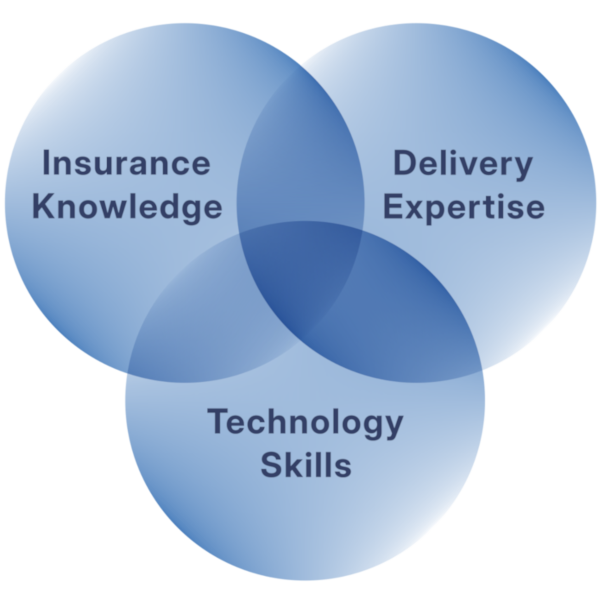